Reconstruction Attacks on Aggressive Relaxations of Differential Privacy
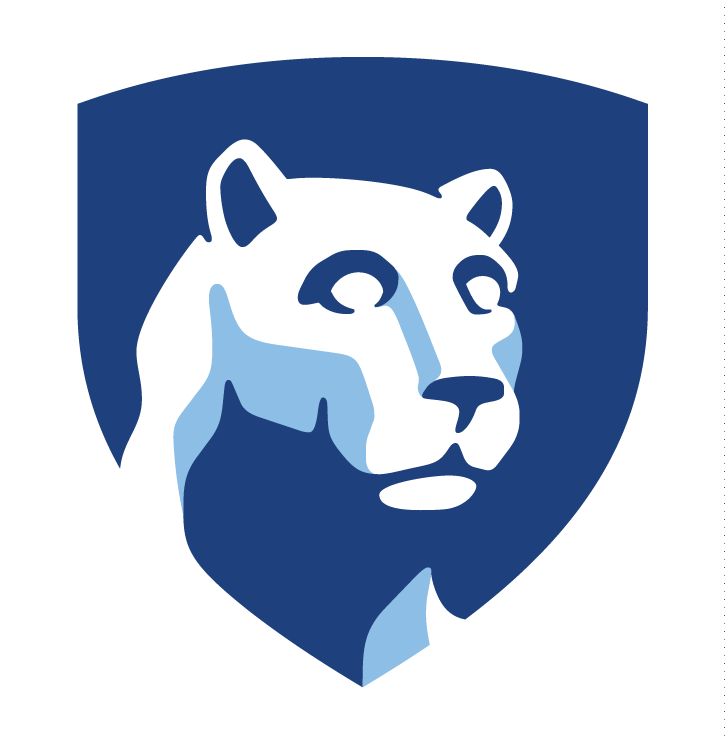
Restricted (Penn State Only)
- Author:
- Durrell, John
- Area of Honors:
- Computer Science
- Degree:
- Bachelor of Science
- Document Type:
- Thesis
- Thesis Supervisors:
- Daniel Kifer, Thesis Supervisor
John Joseph Hannan, Thesis Honors Advisor - Keywords:
- differential privacy
reconstruction attacks
relaxations of differential privacy - Abstract:
- Differential privacy allows statistical agencies to release aggregate information about a dataset while providing formal guarantees about the privacy of individuals whose data may be included. However, despite its widespread support in the privacy community, it has drawn criticism because greater privacy inherently decreases utility for analysts using the dataset. As a result, there is now much ongoing research that attempts to relax the differential privacy definition in search of greater utility. We study a class of relaxations that achieves greater utility by aggressively reducing the set of neighboring pairs whose privacy is protected. While classical differential privacy achieves its privacy guarantees by masking the differences between all possible pairs of datasets that differ by one record, these relaxations choose a definition of neighbors that depends closely on the actual underlying dataset. In particular, we focus on the definitions of individual differential privacy (IDP) and bootstrap differential privacy (BDP), which claim to have similar privacy semantics to classical differential privacy. However, our work demonstrates serious fundamental vulnerabilities in these privacy definitions by presenting attacks against their preferred mechanisms that can reconstruct substantial portions of the dataset at an arbitrarily low privacy cost by the mechanisms' own accounting. These include both large-scale attacks against the entire dataset and small-scale attacks that target information about specific individuals. In fact, we even present queries that extract more information about a dataset when it is protected by these systems than if it was not protected at all. Although we discuss various countermeasures that could defeat these specific attacks, the defenses are either specially tailored to these attacks (and may still be susceptible to other attacks) or are equivalent to simply using differential privacy (thereby negating a relaxed definition's promise of greater utility).